Labeling Tools for Machine Learning: Revolutionizing the Data Annotation Process
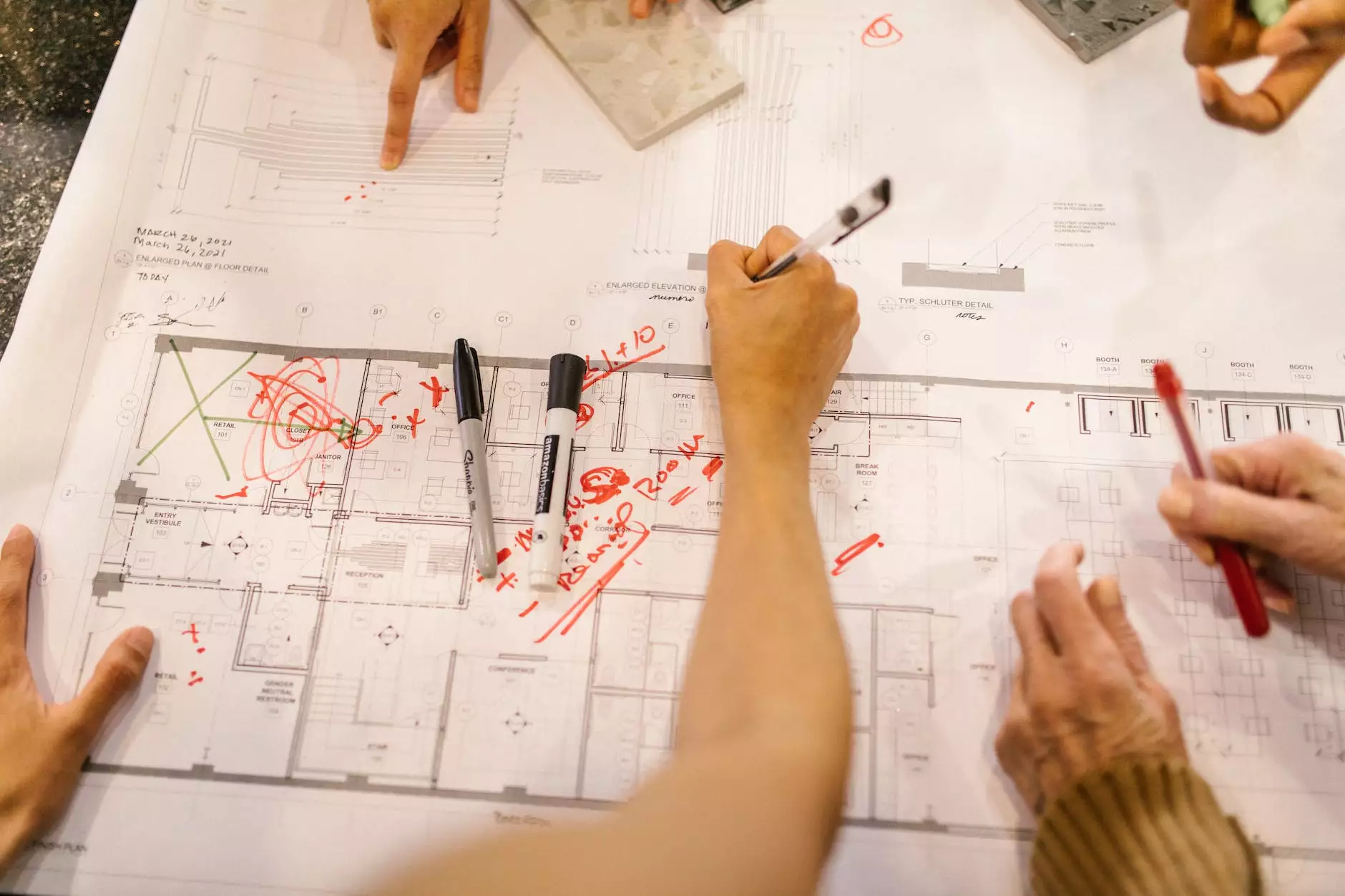
In the modern technological landscape, the significance of labeling tools for machine learning cannot be overstated. These tools serve as the backbone of data annotation, a crucial step in building effective machine learning models. With the exponential growth of data being generated every second, organizations are increasingly turning to advanced labeling tools to streamline their processes and improve their outcomes.
Understanding the Importance of Data Annotation
Data annotation is the process of labeling the data, which is pivotal for supervised learning models. The quality and accuracy of the labeled data directly influence the performance of machine learning algorithms. Here, we'll explore why effective labeling is essential in today's data-driven world.
The Role of Data in Machine Learning
- Foundation of Learning: Machine learning models learn from data. Without accurately labeled datasets, these models cannot make informed predictions or classifications.
- Improves Model Performance: High-quality labeled data leads to better accuracy. The better the data, the more reliable the outcome.
- Facilitates Diverse Applications: From healthcare to finance, labeled data is used across various industries to develop innovative solutions.
Challenges in Data Annotation
Despite its importance, data annotation comes with its challenges. Understanding these obstacles can help organizations navigate the complexities of machine learning better.
- Scalability: As data volumes grow, scaling the annotation process can become cumbersome without appropriate tools.
- Quality Control: Maintaining high standards in data labeling is crucial. Poorly labeled data can lead to flawed machine learning models.
- Time-Consuming tasks: Manual data labeling can be labor-intensive and slow, often leading to delays in project timelines.
The Best Labeling Tools for Machine Learning
To address the challenges of data annotation, various labeling tools have emerged in the market. Below, we discuss some of the leading solutions that significantly enhance the data annotation process.
1. Keymakr: Your Go-To Solution
Keymakr is a pioneering platform that specializes in providing high-quality labeling tools for machine learning. With a focus on user-friendly design and robust functionality, Keymakr empowers businesses in the "Home Services, Keys & Locksmiths" categories to effectively annotate their datasets.
Key Features of Keymakr
- Intuitive Interface: The user-friendly interface allows users to quickly get accustomed to the platform, reducing the learning curve.
- Collaboration Tools: Teams can work together seamlessly, making it easier to manage large projects.
- Quality Assurance: Built-in quality checks ensure that the labeling meets high standards.
- Customizable Solutions: Adaptable to various use cases, making it suitable for diverse industry requirements.
2. Labelbox
Labelbox is another highly regarded tool, specifically designed to support enterprises looking for a scalable annotation solution. With its powerful suite of features, it caters to both simple and complex labeling tasks.
Key Features of Labelbox
- Automation: Labelbox offers automated annotation capabilities that expedite the labeling process.
- Integration: Easily integrates with existing data pipelines, ensuring smooth data flow.
- Team Management: Features that support team collaboration and project tracking.
3. Supervisely
Supervisely is an innovative platform that provides various labeling tools for machine learning, particularly in computer vision projects.
Key Features of Supervisely
- Comprehensive Annotation: Offers sophisticated tools for annotating images and videos, which is vital for visual data applications.
- Collaboration: Provides real-time collaboration features that facilitate teamwork and communication.
- Analytics: Robust analytics to track team productivity and project progress.
How to Choose the Right Labeling Tool for Your Needs
Choosing the right labeling tool for machine learning is critical to the success of your projects. Here are some factors to consider:
1. Project Size and Complexity
Evaluate the scale of your project. For large datasets, you might need a tool that supports scalability and has features for managing big data more efficiently.
2. Types of Data Being Annotated
Consider the types of data involved. If your project requires image or video annotation, ensure the tool has specialized features that cater to those data types.
3. User Experience
The tool should be user-friendly to reduce the learning curve and enhance efficiency. A steep learning curve can slow the project down significantly.
4. Budget
Cost is often a decisive factor. Weigh the pricing of the tool against the features it offers and your overall budget constraint.
Implementation Best Practices When Using Labeling Tools
To maximize the efficacy of labeling tools for machine learning, the following best practices can be applied:
1. Define Clear Guidelines
Establish clear labeling guidelines to ensure consistency and quality across the board. Well-documented protocols can help annotators understand the expectations and reduce ambiguity.
2. Regularly Review Labeling Quality
Implement regular checkpoints to review the quality of annotations. Automated quality checks can help maintain high standards without requiring excessive manual oversight.
3. Train Your Team
Invest in training your team on the selected labeling tool and annotation best practices. The more knowledgeable your team is, the better the quality of the final data will be.
The Future of Labeling Tools in Machine Learning
As machine learning continues to evolve, the tools used for data annotation and labeling will also advance. Here are some future trends to watch:
1. Increased Automation
We can expect significant advancements in automated annotation. Machine learning algorithms themselves will be used more effectively to assist in labeling, thereby reducing the manual workload.
2. Enhanced AI Assistance
Labeling tools will increasingly leverage artificial intelligence to suggest labels based on context and prior examples, making the annotation much faster.
3. Integrated Workflows
Future labeling tools will likely feature more integrations with other data science and machine learning workflows, making end-to-end processes seamless.
Conclusion
The importance of labeling tools for machine learning cannot be overlooked in the quest for developing robust AI solutions. By leveraging tools like Keymakr, organizations can ensure efficient, accurate, and scalable data annotation processes that are essential for training effective machine learning models. As the field evolves, staying informed about the best tools and practices will position your projects for success.
For businesses in the Home Services, Keys & Locksmiths sector, utilizing advanced labeling tools not only enhances operational efficiency but also empowers them to harness the full potential of their data. Stay ahead in the competitive landscape by adopting the right labeling strategies today!