Understanding Image Datasets for Classification
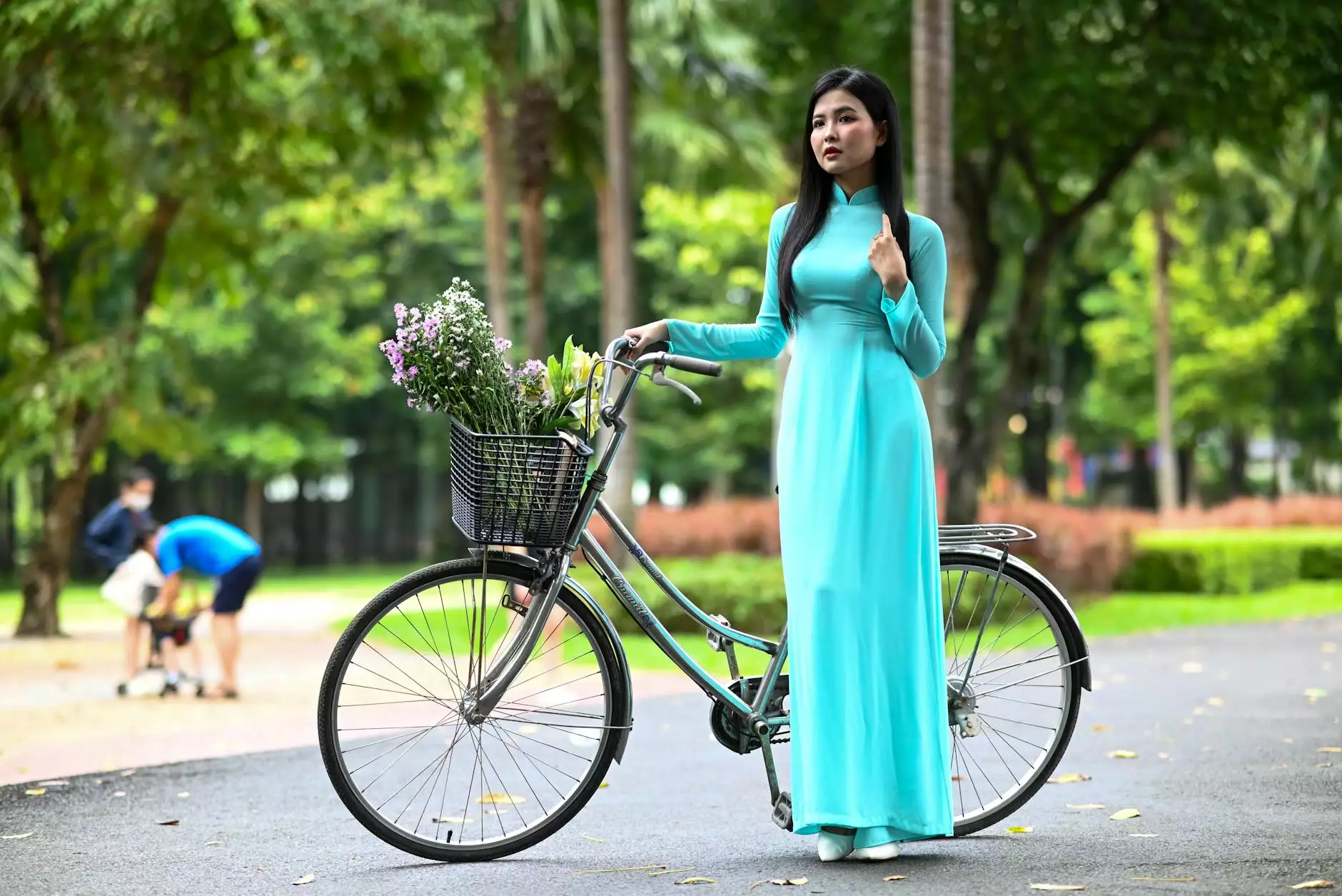
In today’s data-driven world, the importance of image datasets for classification cannot be overstated. Businesses across various sectors leverage these datasets to enhance their AI and machine learning models, particularly in computer vision applications. In this comprehensive guide, we will delve into the intricacies of image datasets for classification, exploring their creation, significance, and the tools available to optimize their effectiveness.
The Importance of Image Datasets in Artificial Intelligence
Image datasets serve as the backbone of any classification task within artificial intelligence (AI). They supply the training data that algorithms need to learn from, helping to teach machines how to identify and categorize various images. In essence, a well-curated image dataset can dramatically improve the performance of a machine learning model.
What Constitutes an Image Dataset?
An image dataset typically comprises a collection of images that are tagged or labeled with information necessary for classification. This tagging process, known as data annotation, involves appending metadata to images that provides context, such as the object class in the image, attributes, and other relevant information.
Types of Image Datasets
- Class-labeled datasets: Datasets where images are categorized into predefined classes.
- Bounding box datasets: These include images with rectangles drawn around objects to indicate their location.
- Semantic segmentation datasets: Datasets where each pixel in the image is labeled, providing more granular classification.
- Instance segmentation datasets: Similar to segmentation datasets but differentiates between distinct instances of the same object class.
Applications of Image Datasets for Classification
The applications of image datasets for classification are vast and span across multiple industries:
1. Healthcare
In the healthcare industry, image classification aids in diagnostic processes. For example, radiology employs image datasets for the classification of X-rays, MRIs, and CT scans, enabling quicker and more accurate diagnostic assessments. Machine learning models trained on these datasets can identify anomalies such as tumors or fractures more effectively than traditional methods.
2. Automotive
Self-driving cars rely heavily on image classification to interpret their surroundings. Datasets containing annotated images of traffic signs, pedestrians, and road conditions are crucial for training autonomous systems to make real-time decisions on the road.
3. Retail
Retailers utilize image datasets to enhance customer experiences. By integrating image recognition technologies, businesses can analyze customer behavior, optimize inventory management, and personalize marketing strategies. For instance, training AI models to recognize products in images can streamline the shopping process for customers.
4. Agriculture
Image datasets in agriculture facilitate precision farming practices. Drones equipped with imaging technologies can collect vast amounts of data from fields, which can be classified using machine learning to assess crop health, predict yields, and identify pest infestations.
Key Considerations for Creating Image Datasets
Developing robust image datasets for classification requires careful planning and implementation. Here are several key considerations:
1. Quality of Images
The quality of images can significantly influence the efficacy of a machine learning model. High-resolution and accurately captured images yield better results. Employing professional photographic techniques can enhance the clarity and detail of the images collected.
2. Diversity and Representativeness
A diverse dataset that represents various scenarios, environments, and conditions ensures that the model will generalize well. Including variations in lighting, angles, and backgrounds can help in training more robust models.
3. Proper Annotation
A meticulous annotation process plays a pivotal role in the quality of the dataset. Using skilled annotators or advanced data annotation tools, such as those provided by KeyLabs.ai, can ensure the accuracy and reliability of labels. This, in turn, enhances model performance and reduces the likelihood of errors during classification.
4. Size of the Dataset
The size of the dataset also matters. Larger datasets can potentially improve the training process by providing the model with more examples to learn from. However, it is essential to balance size with quality—too much irrelevant data can degrade the model’s performance.
How KeyLabs.ai Enhances Data Annotation
At KeyLabs.ai, we specialize in providing exceptional data annotation tools and platforms that streamline the creation of quality image datasets for classification. Here are some ways we enhance the data annotation process:
1. Cutting-edge Technology
Utilizing advanced technologies in data annotation ensures that images are labeled quickly and accurately. Our platform employs AI-assisted annotation tools that help annotators by suggesting labels and identifying potential errors.
2. Scalability
We understand that businesses may need to scale their annotation needs rapidly. KeyLabs.ai offers scalable solutions that can accommodate large-volume projects without compromising on quality.
3. Diverse Annotator Pool
Our platform connects businesses with a diverse pool of skilled annotators around the globe, ensuring that various cultural and contextual nuances are accounted for during the annotation process.
4. Quality Assurance
A stringent quality assurance process is in place to ensure that all annotated data meets the highest standards. Regular audits and feedback loops help maintain the quality of our datasets, crucial for successful machine learning outcomes.
Future Trends in Image Datasets for Classification
The evolution of technology continues to shape the landscape of image datasets for classification. Here are some trends to watch:
1. Increasing Use of Synthetic Data
The generation of synthetic data through advanced techniques such as Generative Adversarial Networks (GANs) is becoming increasingly popular. This approach allows businesses to create large, diverse datasets without the logistical challenges associated with collecting real-world data.
2. Focus on Ethical AI
As AI's role in decision-making grows, the ethical implications of the datasets used are garnering attention. Ensuring diverse and representative datasets is becoming a necessity to prevent bias in machine learning models.
3. Integration of Augmented Reality (AR)
The integration of AR technologies into image datasets could provide new dimensions in terms of data collection and classification. This could include the ability to rapidly annotate images in real-world settings.
Conclusion
In conclusion, image datasets for classification are a fundamental component in the realm of artificial intelligence and machine learning. Businesses across sectors can harness these datasets to unlock the full potential of AI technologies. At KeyLabs.ai, we are committed to providing state-of-the-art data annotation tools and platforms that empower organizations to develop robust models that drive success.
Get Started with KeyLabs.ai
Are you ready to elevate your AI projects with quality image datasets for classification? Explore our data annotation platform today and discover how we can assist you in achieving your business objectives with efficiency and precision.