The Ultimate Guide to Image Labeling Tools for Classification
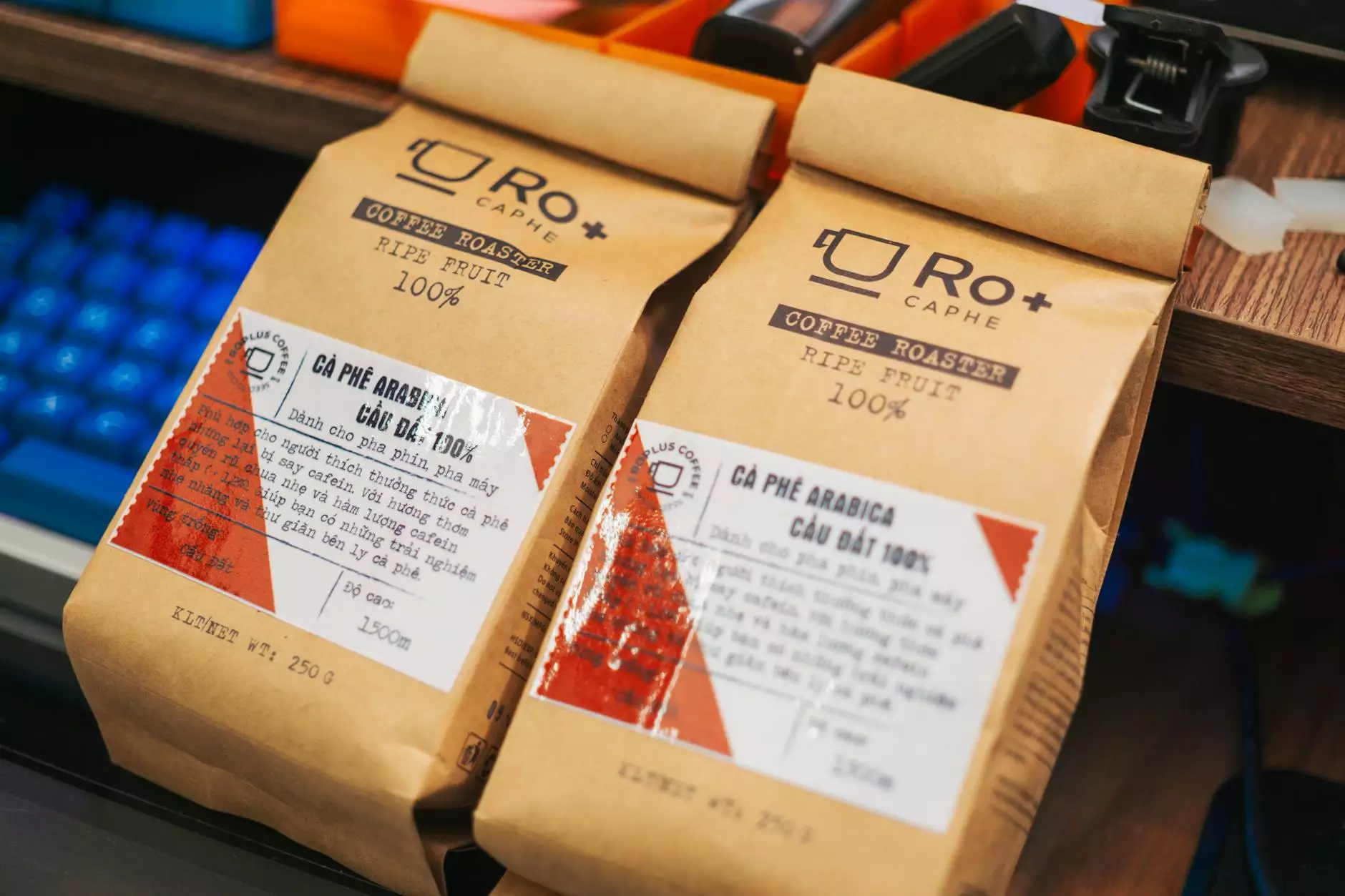
In the era of machine learning and artificial intelligence, data is a crucial asset. With the overwhelming amount of visual data generated every day, image labeling tools for classification have emerged as a critical component in the data annotation landscape. Businesses are increasingly recognizing the importance of utilizing these tools to enhance their data workflows. This comprehensive guide will explore the significance, types, benefits, and best practices of image labeling tools, particularly focusing on their role within the realm of classification.
Understanding Data Annotation and Image Labeling
Before delving into the specifics of image labeling tools, it is vital to understand data annotation. Data annotation is the process of labeling datasets to make them understandable for machine learning algorithms. It transforms raw data into a format that can be analyzed effectively. This is particularly important for tasks involving computer vision, where images must be tagged accurately in order for models to learn and generalize from those examples.
Image labeling is a crucial subset of data annotation, focusing specifically on visual data. In the context of machine learning, it typically involves tagging images with labels that describe their content. These labels can be categories, objects, or features within the image, providing the necessary context for algorithms to classify or recognize various elements. Utilizing an image labeling tool for classification streamlines this process, allowing for more efficient and accurate data preparation.
Why Use an Image Labeling Tool?
The advantages of employing an image labeling tool for classification are numerous:
- Increased Efficiency: Automated tools can significantly speed up the labeling process compared to manual annotation, enabling businesses to handle larger datasets in shorter timeframes.
- Improved Accuracy: Advanced image labeling tools leverage machine learning and artificial intelligence to reduce human error, enhancing the overall quality of the labeled data.
- Scalability: As data volumes grow, labeling tools provide the scalability necessary to maintain productivity without sacrificing quality.
- Cost-Effectiveness: Automating the labeling process can reduce labor costs associated with manual data annotation while also accelerating project timelines.
- User-Friendly Interfaces: Modern image labeling tools are designed with intuitive interfaces that facilitate easy use, even for those without extensive technical expertise.
Types of Image Labeling Tools for Classification
There are several types of image labeling tools, each offering unique features suited for different use cases:
1. Open Source Tools
Open-source image labeling tools are free to use and can be modified to fit specific needs. Examples include:
- LabelImg: A graphical image annotation tool for labeling object bounding boxes in images.
- VGG Image Annotator (VIA): A simple and standalone manual annotation tool that runs in a web browser.
2. Commercial Tools
Commercial tools often provide more features, support, and advanced capabilities. Notable examples include:
- Keylabs.ai: A leading data annotation platform that offers robust solutions for image labeling and classification. Its user-friendly interface and powerful labeling capabilities set it apart.
- SuperAnnotate: An end-to-end image annotation tool designed to handle complex labeling tasks with ease.
3. Cloud-Based Tools
Cloud-based image labeling tools facilitate easy access from any internet-enabled device. They support collaborative efforts and provide scalable storage solutions. Noteworthy tools include:
- Amazon SageMaker Ground Truth: A managed data labeling service that uses machine learning to speed up the creation of labeled datasets.
- Labelbox: A flexible platform that allows teams to manage the entire data labeling process efficiently.
Choosing the Right Image Labeling Tool
Selecting an image labeling tool for classification is crucial for ensuring the success of your machine learning projects. Here are key factors to consider:
- Project Requirements: Assess the specific needs of your project. Consider the type and volume of images, as well as the complexity of labeling required.
- User Experience: Opt for a tool with an intuitive interface that can be easily understood by all stakeholders involved in the labeling process.
- Integration Capabilities: Ensure that the tool can integrate with your existing workflow, data management systems, and machine learning frameworks.
- Support and Resources: Choose a tool backed by robust customer support and comprehensive resources, including tutorials and community forums.
- Cost: Consider your budget and the overall return on investment. Weigh the features offered against your requirements.
Best Practices for Using Image Labeling Tools
To leverage the full potential of image labeling tools for classification, adhere to the following best practices:
1. Define Clear Guidelines
Establish a set of clear annotation guidelines to ensure consistency across the labeled data. This is essential for maintaining the quality of the dataset, especially when multiple annotators are involved.
2. Leverage Active Learning
Utilize active learning strategies to identify uncertain predictions and focus human labeling efforts where they are most needed. This can dramatically improve the efficiency of the labeling process.
3. Review and Validate
Regularly review the labeled images to validate the accuracy of annotations. Implement a feedback loop to continuously improve the annotation process.
4. Train Annotators
Provide training and resources for annotators to familiarize them with the tool and the project requirements. Well-trained annotators can significantly enhance the quality and speed of the labeling process.
5. Utilize Quality Control Measures
Implement quality control measures such as redundancy in labeling (having multiple annotators label the same data) to ensure the highest level of accuracy in your dataset.
Conclusion: Transforming Your Data Annotation Process
The rise of image labeling tools for classification represents a significant advancement in the field of data annotation. Businesses that adopt these tools can enhance their efficiency, accuracy, and scalability while driving better outcomes for machine learning applications. Investing in a robust imaging labeling solution like Keylabs.ai can fundamentally transform your data annotation process and empower your organization to harness the full potential of its visual data.
With the right tools and best practices in place, the future of data annotation looks remarkably promising, opening up new avenues for innovation and success in an increasingly data-driven world.